- Enterprise AI Solutions
- Posts
- Legal Whiplash & Learning Machines
Legal Whiplash & Learning Machines
As AI regulation mutates and models get meta, strategy must move faster than policy—and smarter than the lab

TGIF!
If your AI strategy still assumes legal clarity, stable governance, or a six-month planning window, you may be overdue for an upgrade.
Today we’re talking about the shifting legal terrain enterprises must now navigate, a new “periodic table” for machine learning discovery, Apple’s internal AI reorg drama, and OpenAI’s next play: giving their models the power to call other models for help.
Let’s get into it.
Start learning AI in 2025
Keeping up with AI is hard – we get it!
That’s why over 1M professionals read Superhuman AI to stay ahead.
Get daily AI news, tools, and tutorials
Learn new AI skills you can use at work in 3 mins a day
Become 10X more productive
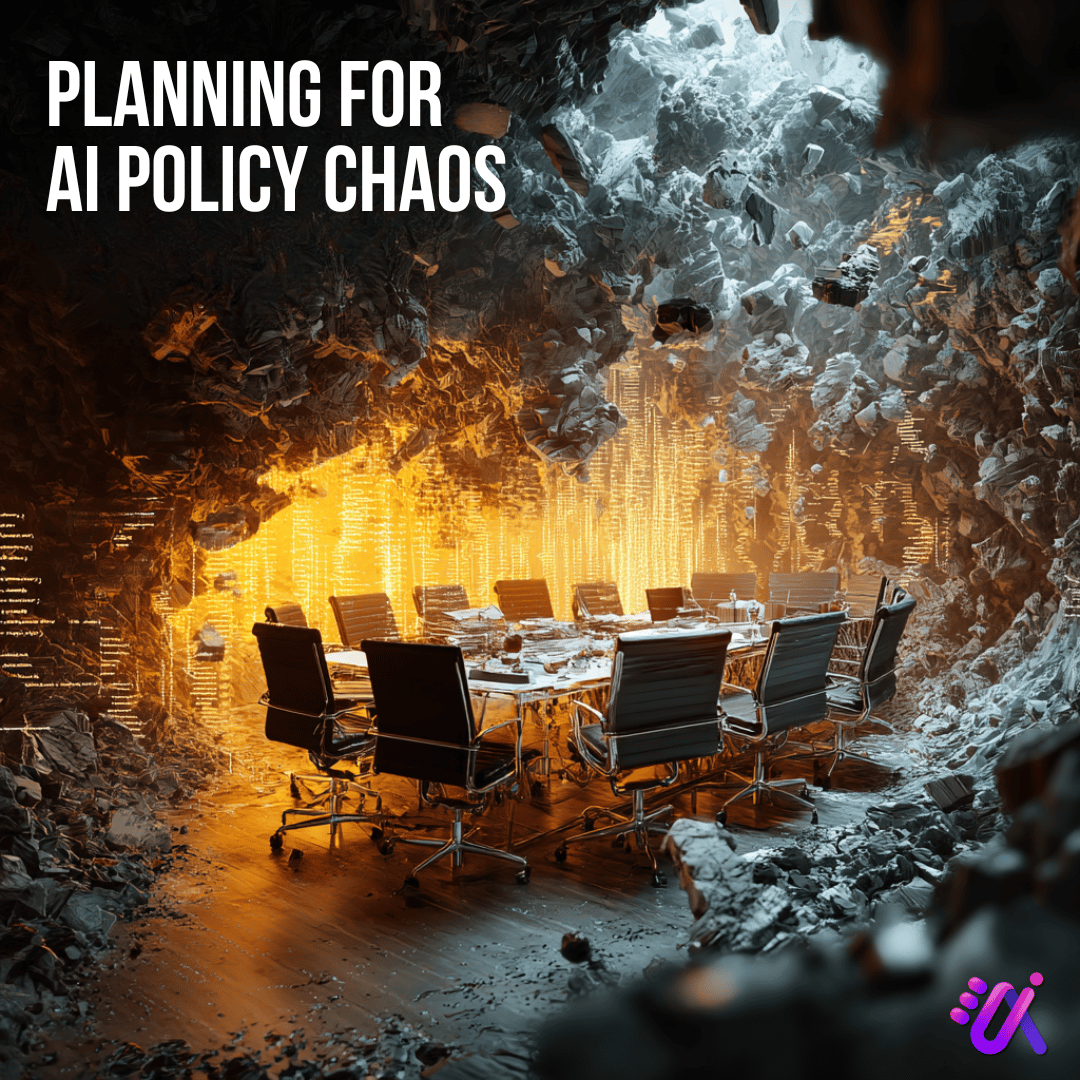
Enterprise AI Solutions
Building AI Strategy in a Shifting Legal Landscape
AI is both evolving faster than our workflows, and is outpacing the legal frameworks meant to contain it.
Here’s what’s making this moment particularly volatile:
Regulations are being drafted in real-time, and often retroactively.
Vendors are releasing features without full legal vetting, leaving enterprises to absorb the fallout.
New liabilities are emerging from edge-case AI behavior, especially in hiring, finance, and healthcare.
If your AI strategy assumes a stable compliance environment, you’re building on quicksand.
What to do instead:
Adopt regulatory agility. Build multiple legal scenarios (Plan A, B, C) into your deployment roadmap.
Build compliance into your vendor vetting process, not just product evaluation.
Create a “legal readiness” dashboard that includes model transparency, explainability protocols, and usage audit trails.
Bottom line: legal risk is now a competitive differentiator. The companies who plan for policy chaos are the ones who won’t have to pause mid-scale to clean up PR disasters.
What to Watch: A Periodic Table for Machine Learning
No, this isn’t chemistry class—it’s next-gen AI development.
MIT researchers just released a “periodic table” for machine learning, organizing ML techniques like atoms to make them easier to combine, iterate on, and discover new patterns.
Why this matters:
It formalizes ML as a modular design system—opening the door for AI “engineering,” not just experimentation.
It gives enterprises a way to evaluate and recombine existing architectures instead of blindly chasing model-of-the-week.
It could accelerate internal R&D by helping teams innovate on top of established building blocks.
Think of it as Lego bricks for your data science team’s next breakthrough.

In the News: Models, Meta-Moves, and Apple’s AI Shuffle
Apple reorgs its secret robotics team—again.
The AI chief who took over Siri? No longer managing Apple’s robotics lab. Internal reshuffling signals a possible shift in AI priorities—away from sci-fi hardware and back toward near-term software UX.
Full scoop →OpenAI wants its models to call other models.
OpenAI is working on tech that lets one AI model invoke another one as a “helper.” Think chain-of-thought reasoning meets cloud orchestration. Enterprise lesson: composable AI is no longer theoretical—it’s shipping.
Read more →Nature warns: science isn’t keeping up with AI.
As AI races ahead, scientific research is struggling to keep pace with its implications. From reproducibility to peer review, the systems built to validate progress are being outpaced by the models themselves.
Full re →
TL;DR:
AI strategy can’t wait for regulation.
MIT’s new ML framework could help your team build faster, better models using proven components.
Apple’s internal AI shuffle hints at a UX-first pivot.
OpenAI is designing AI that builds AI—automatically.
Even science is scrambling to catch up. If your org isn’t sprinting, it’s standing still.
Stay sharp,
Cat Valverde
Founder, Enterprise AI Solutions
Navigating Tomorrow’s Tech Landscape Together
How'd we do?Let us know how this newsletter landed for you. |